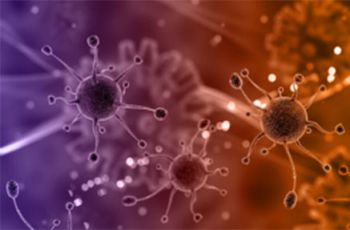
Online
Janette Rodrigues, Administrative Director, Office of Integrative Medicine & Health
jrodrigues [at] gwu [dot] edu (jrodrigues[at]gwu[dot]edu)
Ali Rahnavard, PhD, Assistant Professor of Biostatistics and Bioinformatics, GW Milken Institute School of Public Health, is giving a free online talk on "Decoding Metabolomics Data to Infer Biological Insights" as part of the GW Biomedical Cross-disciplinary Seminar Series sponsored by the Office of Integrative Medicine & Health and the Department of Biochemistry & Molecular Medicine at the GW School of Medicine & Health Sciences.
Abstract
A deep functional understanding of the molecular pathways that mediate post-treatment health improvements holds significant promise to lead to new therapeutic strategies for complex metabolic diseases. In this talk, we introduce three novel computational tools for generic metabolomics data analysis.
- Tweedieverse for differential analysis, rooted in Tweedie generalized linear models, is tailored to address the unique statistical properties of metabolomics data, accommodating variations both within and between experimental platforms.
- MassSight enables LC-MS data harmonization, including combing metabolite profiles using peak alignment and data consolidation.
- Waveome complements these tools with its adaptable machine learning techniques, offering a user-friendly approach to dissect longitudinal metabolomics data and thus allowing for an enriched understanding of metabolite dynamics crucial for drug discovery and metabolic research.
In the context of these advancements, we discuss the improvements of two distinct treatments:
- Obesity and type 2 diabetes following Roux-en-Y gastric bypass (RYGB), and:
- Type 1 Neurofibromatosis cancer post-MEKi treatment. Using time-course integrated proteomics and metabolomics analysis, we identified potential circulating factors that mediate the weight loss-independent therapeutic benefits of RYGB and characterize functions that led to cancer improvement. Using animal experiments coupled with functional enrichment and integrative analyses, we identified and validated metabolic pathways underlying biological pathways of these treatments.